GUIDE to Worksheet 2.3
Contents
GUIDE to Worksheet 2.3#
This notebook is meant to provide hints and guidance on how to complete Worksheet 2.3: Bootstrapping linear regression. It will not necessarily answer every part of every problem, but it will get you to the interesting points of the worksheet.
Also, these guides may be useful for you as you are building up your coding toolkit to see different ways to execute different tasks in Python. I am not necessarily showing the most efficient or elegant code, but trying to illustrate different ways to do things. You should always use the code you feel you can understand best.
First, let’s take care of our imported modules.
import matplotlib.pyplot as plt
import numpy as np
import scipy.stats as st
import seaborn as sns
from sklearn.linear_model import LinearRegression as skLinReg
sns.set(color_codes=True)
%matplotlib inline
In this worksheet, you will be working with the linked data set, which is described here.
We will be performing estimation of the model parameters \(\beta_0\), \(\beta_3\), and \(\beta_6\) in the model:
That is, we think that the first measurement in each observation depends linearly on the 4th and 7th. It will be up to you to use the metadata to learn what these measurements are.
At the end of this worksheet, you should have a figure that shows the model, the data, the parameter estimates, and the spread in those parameter estimates. This can be one panel or many, but it should be one easy-to-understand figure that has all of those components.
Part 1#
Use the open
and file.readlines
functions to open the data set. How does it seem that the measurements in each line of the data are organized? What type of data are the lines? How can we split
the lines and grab the measurements we want (1st, 4th, and 7th columns)? How can we do this repeatedly for all lines of the data? You may also have to check the data for missing or invalid values!
Let’s first try and open the data and see what we’re dealing with. The first thing we can try, although it’s not always useful, is to simply use open
and file.readlines
or file.read
to see everything in raw ascii.
mpgData = "auto-mpg.data"
mpgNames = "auto-mpg.names"
with open("./Resources/" + mpgNames, 'r') as f:
print(f.read()) ## Try f.readlines() and see what happens!
1. Title: Auto-Mpg Data
2. Sources:
(a) Origin: This dataset was taken from the StatLib library which is
maintained at Carnegie Mellon University. The dataset was
used in the 1983 American Statistical Association Exposition.
(c) Date: July 7, 1993
3. Past Usage:
- See 2b (above)
- Quinlan,R. (1993). Combining Instance-Based and Model-Based Learning.
In Proceedings on the Tenth International Conference of Machine
Learning, 236-243, University of Massachusetts, Amherst. Morgan
Kaufmann.
4. Relevant Information:
This dataset is a slightly modified version of the dataset provided in
the StatLib library. In line with the use by Ross Quinlan (1993) in
predicting the attribute "mpg", 8 of the original instances were removed
because they had unknown values for the "mpg" attribute. The original
dataset is available in the file "auto-mpg.data-original".
"The data concerns city-cycle fuel consumption in miles per gallon,
to be predicted in terms of 3 multivalued discrete and 5 continuous
attributes." (Quinlan, 1993)
5. Number of Instances: 398
6. Number of Attributes: 9 including the class attribute
7. Attribute Information:
1. mpg: continuous
2. cylinders: multi-valued discrete
3. displacement: continuous
4. horsepower: continuous
5. weight: continuous
6. acceleration: continuous
7. model year: multi-valued discrete
8. origin: multi-valued discrete
9. car name: string (unique for each instance)
8. Missing Attribute Values: horsepower has 6 missing values
We can see that the auto-mpg.names
file contains a description of the data set! This means that the model we want to explore is the linear relationship between horsepower and model year and mpg.
Let’s look at the auto-mpg.data
now.
with open("./Resources/" + mpgData, 'r') as f:
print(f.read())
18.0 8 307.0 130.0 3504. 12.0 70 1 "chevrolet chevelle malibu"
15.0 8 350.0 165.0 3693. 11.5 70 1 "buick skylark 320"
18.0 8 318.0 150.0 3436. 11.0 70 1 "plymouth satellite"
16.0 8 304.0 150.0 3433. 12.0 70 1 "amc rebel sst"
17.0 8 302.0 140.0 3449. 10.5 70 1 "ford torino"
15.0 8 429.0 198.0 4341. 10.0 70 1 "ford galaxie 500"
14.0 8 454.0 220.0 4354. 9.0 70 1 "chevrolet impala"
14.0 8 440.0 215.0 4312. 8.5 70 1 "plymouth fury iii"
14.0 8 455.0 225.0 4425. 10.0 70 1 "pontiac catalina"
15.0 8 390.0 190.0 3850. 8.5 70 1 "amc ambassador dpl"
15.0 8 383.0 170.0 3563. 10.0 70 1 "dodge challenger se"
14.0 8 340.0 160.0 3609. 8.0 70 1 "plymouth 'cuda 340"
15.0 8 400.0 150.0 3761. 9.5 70 1 "chevrolet monte carlo"
14.0 8 455.0 225.0 3086. 10.0 70 1 "buick estate wagon (sw)"
24.0 4 113.0 95.00 2372. 15.0 70 3 "toyota corona mark ii"
22.0 6 198.0 95.00 2833. 15.5 70 1 "plymouth duster"
18.0 6 199.0 97.00 2774. 15.5 70 1 "amc hornet"
21.0 6 200.0 85.00 2587. 16.0 70 1 "ford maverick"
27.0 4 97.00 88.00 2130. 14.5 70 3 "datsun pl510"
26.0 4 97.00 46.00 1835. 20.5 70 2 "volkswagen 1131 deluxe sedan"
25.0 4 110.0 87.00 2672. 17.5 70 2 "peugeot 504"
24.0 4 107.0 90.00 2430. 14.5 70 2 "audi 100 ls"
25.0 4 104.0 95.00 2375. 17.5 70 2 "saab 99e"
26.0 4 121.0 113.0 2234. 12.5 70 2 "bmw 2002"
21.0 6 199.0 90.00 2648. 15.0 70 1 "amc gremlin"
10.0 8 360.0 215.0 4615. 14.0 70 1 "ford f250"
10.0 8 307.0 200.0 4376. 15.0 70 1 "chevy c20"
11.0 8 318.0 210.0 4382. 13.5 70 1 "dodge d200"
9.0 8 304.0 193.0 4732. 18.5 70 1 "hi 1200d"
27.0 4 97.00 88.00 2130. 14.5 71 3 "datsun pl510"
28.0 4 140.0 90.00 2264. 15.5 71 1 "chevrolet vega 2300"
25.0 4 113.0 95.00 2228. 14.0 71 3 "toyota corona"
25.0 4 98.00 ? 2046. 19.0 71 1 "ford pinto"
19.0 6 232.0 100.0 2634. 13.0 71 1 "amc gremlin"
16.0 6 225.0 105.0 3439. 15.5 71 1 "plymouth satellite custom"
17.0 6 250.0 100.0 3329. 15.5 71 1 "chevrolet chevelle malibu"
19.0 6 250.0 88.00 3302. 15.5 71 1 "ford torino 500"
18.0 6 232.0 100.0 3288. 15.5 71 1 "amc matador"
14.0 8 350.0 165.0 4209. 12.0 71 1 "chevrolet impala"
14.0 8 400.0 175.0 4464. 11.5 71 1 "pontiac catalina brougham"
14.0 8 351.0 153.0 4154. 13.5 71 1 "ford galaxie 500"
14.0 8 318.0 150.0 4096. 13.0 71 1 "plymouth fury iii"
12.0 8 383.0 180.0 4955. 11.5 71 1 "dodge monaco (sw)"
13.0 8 400.0 170.0 4746. 12.0 71 1 "ford country squire (sw)"
13.0 8 400.0 175.0 5140. 12.0 71 1 "pontiac safari (sw)"
18.0 6 258.0 110.0 2962. 13.5 71 1 "amc hornet sportabout (sw)"
22.0 4 140.0 72.00 2408. 19.0 71 1 "chevrolet vega (sw)"
19.0 6 250.0 100.0 3282. 15.0 71 1 "pontiac firebird"
18.0 6 250.0 88.00 3139. 14.5 71 1 "ford mustang"
23.0 4 122.0 86.00 2220. 14.0 71 1 "mercury capri 2000"
28.0 4 116.0 90.00 2123. 14.0 71 2 "opel 1900"
30.0 4 79.00 70.00 2074. 19.5 71 2 "peugeot 304"
30.0 4 88.00 76.00 2065. 14.5 71 2 "fiat 124b"
31.0 4 71.00 65.00 1773. 19.0 71 3 "toyota corolla 1200"
35.0 4 72.00 69.00 1613. 18.0 71 3 "datsun 1200"
27.0 4 97.00 60.00 1834. 19.0 71 2 "volkswagen model 111"
26.0 4 91.00 70.00 1955. 20.5 71 1 "plymouth cricket"
24.0 4 113.0 95.00 2278. 15.5 72 3 "toyota corona hardtop"
25.0 4 97.50 80.00 2126. 17.0 72 1 "dodge colt hardtop"
23.0 4 97.00 54.00 2254. 23.5 72 2 "volkswagen type 3"
20.0 4 140.0 90.00 2408. 19.5 72 1 "chevrolet vega"
21.0 4 122.0 86.00 2226. 16.5 72 1 "ford pinto runabout"
13.0 8 350.0 165.0 4274. 12.0 72 1 "chevrolet impala"
14.0 8 400.0 175.0 4385. 12.0 72 1 "pontiac catalina"
15.0 8 318.0 150.0 4135. 13.5 72 1 "plymouth fury iii"
14.0 8 351.0 153.0 4129. 13.0 72 1 "ford galaxie 500"
17.0 8 304.0 150.0 3672. 11.5 72 1 "amc ambassador sst"
11.0 8 429.0 208.0 4633. 11.0 72 1 "mercury marquis"
13.0 8 350.0 155.0 4502. 13.5 72 1 "buick lesabre custom"
12.0 8 350.0 160.0 4456. 13.5 72 1 "oldsmobile delta 88 royale"
13.0 8 400.0 190.0 4422. 12.5 72 1 "chrysler newport royal"
19.0 3 70.00 97.00 2330. 13.5 72 3 "mazda rx2 coupe"
15.0 8 304.0 150.0 3892. 12.5 72 1 "amc matador (sw)"
13.0 8 307.0 130.0 4098. 14.0 72 1 "chevrolet chevelle concours (sw)"
13.0 8 302.0 140.0 4294. 16.0 72 1 "ford gran torino (sw)"
14.0 8 318.0 150.0 4077. 14.0 72 1 "plymouth satellite custom (sw)"
18.0 4 121.0 112.0 2933. 14.5 72 2 "volvo 145e (sw)"
22.0 4 121.0 76.00 2511. 18.0 72 2 "volkswagen 411 (sw)"
21.0 4 120.0 87.00 2979. 19.5 72 2 "peugeot 504 (sw)"
26.0 4 96.00 69.00 2189. 18.0 72 2 "renault 12 (sw)"
22.0 4 122.0 86.00 2395. 16.0 72 1 "ford pinto (sw)"
28.0 4 97.00 92.00 2288. 17.0 72 3 "datsun 510 (sw)"
23.0 4 120.0 97.00 2506. 14.5 72 3 "toyouta corona mark ii (sw)"
28.0 4 98.00 80.00 2164. 15.0 72 1 "dodge colt (sw)"
27.0 4 97.00 88.00 2100. 16.5 72 3 "toyota corolla 1600 (sw)"
13.0 8 350.0 175.0 4100. 13.0 73 1 "buick century 350"
14.0 8 304.0 150.0 3672. 11.5 73 1 "amc matador"
13.0 8 350.0 145.0 3988. 13.0 73 1 "chevrolet malibu"
14.0 8 302.0 137.0 4042. 14.5 73 1 "ford gran torino"
15.0 8 318.0 150.0 3777. 12.5 73 1 "dodge coronet custom"
12.0 8 429.0 198.0 4952. 11.5 73 1 "mercury marquis brougham"
13.0 8 400.0 150.0 4464. 12.0 73 1 "chevrolet caprice classic"
13.0 8 351.0 158.0 4363. 13.0 73 1 "ford ltd"
14.0 8 318.0 150.0 4237. 14.5 73 1 "plymouth fury gran sedan"
13.0 8 440.0 215.0 4735. 11.0 73 1 "chrysler new yorker brougham"
12.0 8 455.0 225.0 4951. 11.0 73 1 "buick electra 225 custom"
13.0 8 360.0 175.0 3821. 11.0 73 1 "amc ambassador brougham"
18.0 6 225.0 105.0 3121. 16.5 73 1 "plymouth valiant"
16.0 6 250.0 100.0 3278. 18.0 73 1 "chevrolet nova custom"
18.0 6 232.0 100.0 2945. 16.0 73 1 "amc hornet"
18.0 6 250.0 88.00 3021. 16.5 73 1 "ford maverick"
23.0 6 198.0 95.00 2904. 16.0 73 1 "plymouth duster"
26.0 4 97.00 46.00 1950. 21.0 73 2 "volkswagen super beetle"
11.0 8 400.0 150.0 4997. 14.0 73 1 "chevrolet impala"
12.0 8 400.0 167.0 4906. 12.5 73 1 "ford country"
13.0 8 360.0 170.0 4654. 13.0 73 1 "plymouth custom suburb"
12.0 8 350.0 180.0 4499. 12.5 73 1 "oldsmobile vista cruiser"
18.0 6 232.0 100.0 2789. 15.0 73 1 "amc gremlin"
20.0 4 97.00 88.00 2279. 19.0 73 3 "toyota carina"
21.0 4 140.0 72.00 2401. 19.5 73 1 "chevrolet vega"
22.0 4 108.0 94.00 2379. 16.5 73 3 "datsun 610"
18.0 3 70.00 90.00 2124. 13.5 73 3 "maxda rx3"
19.0 4 122.0 85.00 2310. 18.5 73 1 "ford pinto"
21.0 6 155.0 107.0 2472. 14.0 73 1 "mercury capri v6"
26.0 4 98.00 90.00 2265. 15.5 73 2 "fiat 124 sport coupe"
15.0 8 350.0 145.0 4082. 13.0 73 1 "chevrolet monte carlo s"
16.0 8 400.0 230.0 4278. 9.50 73 1 "pontiac grand prix"
29.0 4 68.00 49.00 1867. 19.5 73 2 "fiat 128"
24.0 4 116.0 75.00 2158. 15.5 73 2 "opel manta"
20.0 4 114.0 91.00 2582. 14.0 73 2 "audi 100ls"
19.0 4 121.0 112.0 2868. 15.5 73 2 "volvo 144ea"
15.0 8 318.0 150.0 3399. 11.0 73 1 "dodge dart custom"
24.0 4 121.0 110.0 2660. 14.0 73 2 "saab 99le"
20.0 6 156.0 122.0 2807. 13.5 73 3 "toyota mark ii"
11.0 8 350.0 180.0 3664. 11.0 73 1 "oldsmobile omega"
20.0 6 198.0 95.00 3102. 16.5 74 1 "plymouth duster"
21.0 6 200.0 ? 2875. 17.0 74 1 "ford maverick"
19.0 6 232.0 100.0 2901. 16.0 74 1 "amc hornet"
15.0 6 250.0 100.0 3336. 17.0 74 1 "chevrolet nova"
31.0 4 79.00 67.00 1950. 19.0 74 3 "datsun b210"
26.0 4 122.0 80.00 2451. 16.5 74 1 "ford pinto"
32.0 4 71.00 65.00 1836. 21.0 74 3 "toyota corolla 1200"
25.0 4 140.0 75.00 2542. 17.0 74 1 "chevrolet vega"
16.0 6 250.0 100.0 3781. 17.0 74 1 "chevrolet chevelle malibu classic"
16.0 6 258.0 110.0 3632. 18.0 74 1 "amc matador"
18.0 6 225.0 105.0 3613. 16.5 74 1 "plymouth satellite sebring"
16.0 8 302.0 140.0 4141. 14.0 74 1 "ford gran torino"
13.0 8 350.0 150.0 4699. 14.5 74 1 "buick century luxus (sw)"
14.0 8 318.0 150.0 4457. 13.5 74 1 "dodge coronet custom (sw)"
14.0 8 302.0 140.0 4638. 16.0 74 1 "ford gran torino (sw)"
14.0 8 304.0 150.0 4257. 15.5 74 1 "amc matador (sw)"
29.0 4 98.00 83.00 2219. 16.5 74 2 "audi fox"
26.0 4 79.00 67.00 1963. 15.5 74 2 "volkswagen dasher"
26.0 4 97.00 78.00 2300. 14.5 74 2 "opel manta"
31.0 4 76.00 52.00 1649. 16.5 74 3 "toyota corona"
32.0 4 83.00 61.00 2003. 19.0 74 3 "datsun 710"
28.0 4 90.00 75.00 2125. 14.5 74 1 "dodge colt"
24.0 4 90.00 75.00 2108. 15.5 74 2 "fiat 128"
26.0 4 116.0 75.00 2246. 14.0 74 2 "fiat 124 tc"
24.0 4 120.0 97.00 2489. 15.0 74 3 "honda civic"
26.0 4 108.0 93.00 2391. 15.5 74 3 "subaru"
31.0 4 79.00 67.00 2000. 16.0 74 2 "fiat x1.9"
19.0 6 225.0 95.00 3264. 16.0 75 1 "plymouth valiant custom"
18.0 6 250.0 105.0 3459. 16.0 75 1 "chevrolet nova"
15.0 6 250.0 72.00 3432. 21.0 75 1 "mercury monarch"
15.0 6 250.0 72.00 3158. 19.5 75 1 "ford maverick"
16.0 8 400.0 170.0 4668. 11.5 75 1 "pontiac catalina"
15.0 8 350.0 145.0 4440. 14.0 75 1 "chevrolet bel air"
16.0 8 318.0 150.0 4498. 14.5 75 1 "plymouth grand fury"
14.0 8 351.0 148.0 4657. 13.5 75 1 "ford ltd"
17.0 6 231.0 110.0 3907. 21.0 75 1 "buick century"
16.0 6 250.0 105.0 3897. 18.5 75 1 "chevroelt chevelle malibu"
15.0 6 258.0 110.0 3730. 19.0 75 1 "amc matador"
18.0 6 225.0 95.00 3785. 19.0 75 1 "plymouth fury"
21.0 6 231.0 110.0 3039. 15.0 75 1 "buick skyhawk"
20.0 8 262.0 110.0 3221. 13.5 75 1 "chevrolet monza 2+2"
13.0 8 302.0 129.0 3169. 12.0 75 1 "ford mustang ii"
29.0 4 97.00 75.00 2171. 16.0 75 3 "toyota corolla"
23.0 4 140.0 83.00 2639. 17.0 75 1 "ford pinto"
20.0 6 232.0 100.0 2914. 16.0 75 1 "amc gremlin"
23.0 4 140.0 78.00 2592. 18.5 75 1 "pontiac astro"
24.0 4 134.0 96.00 2702. 13.5 75 3 "toyota corona"
25.0 4 90.00 71.00 2223. 16.5 75 2 "volkswagen dasher"
24.0 4 119.0 97.00 2545. 17.0 75 3 "datsun 710"
18.0 6 171.0 97.00 2984. 14.5 75 1 "ford pinto"
29.0 4 90.00 70.00 1937. 14.0 75 2 "volkswagen rabbit"
19.0 6 232.0 90.00 3211. 17.0 75 1 "amc pacer"
23.0 4 115.0 95.00 2694. 15.0 75 2 "audi 100ls"
23.0 4 120.0 88.00 2957. 17.0 75 2 "peugeot 504"
22.0 4 121.0 98.00 2945. 14.5 75 2 "volvo 244dl"
25.0 4 121.0 115.0 2671. 13.5 75 2 "saab 99le"
33.0 4 91.00 53.00 1795. 17.5 75 3 "honda civic cvcc"
28.0 4 107.0 86.00 2464. 15.5 76 2 "fiat 131"
25.0 4 116.0 81.00 2220. 16.9 76 2 "opel 1900"
25.0 4 140.0 92.00 2572. 14.9 76 1 "capri ii"
26.0 4 98.00 79.00 2255. 17.7 76 1 "dodge colt"
27.0 4 101.0 83.00 2202. 15.3 76 2 "renault 12tl"
17.5 8 305.0 140.0 4215. 13.0 76 1 "chevrolet chevelle malibu classic"
16.0 8 318.0 150.0 4190. 13.0 76 1 "dodge coronet brougham"
15.5 8 304.0 120.0 3962. 13.9 76 1 "amc matador"
14.5 8 351.0 152.0 4215. 12.8 76 1 "ford gran torino"
22.0 6 225.0 100.0 3233. 15.4 76 1 "plymouth valiant"
22.0 6 250.0 105.0 3353. 14.5 76 1 "chevrolet nova"
24.0 6 200.0 81.00 3012. 17.6 76 1 "ford maverick"
22.5 6 232.0 90.00 3085. 17.6 76 1 "amc hornet"
29.0 4 85.00 52.00 2035. 22.2 76 1 "chevrolet chevette"
24.5 4 98.00 60.00 2164. 22.1 76 1 "chevrolet woody"
29.0 4 90.00 70.00 1937. 14.2 76 2 "vw rabbit"
33.0 4 91.00 53.00 1795. 17.4 76 3 "honda civic"
20.0 6 225.0 100.0 3651. 17.7 76 1 "dodge aspen se"
18.0 6 250.0 78.00 3574. 21.0 76 1 "ford granada ghia"
18.5 6 250.0 110.0 3645. 16.2 76 1 "pontiac ventura sj"
17.5 6 258.0 95.00 3193. 17.8 76 1 "amc pacer d/l"
29.5 4 97.00 71.00 1825. 12.2 76 2 "volkswagen rabbit"
32.0 4 85.00 70.00 1990. 17.0 76 3 "datsun b-210"
28.0 4 97.00 75.00 2155. 16.4 76 3 "toyota corolla"
26.5 4 140.0 72.00 2565. 13.6 76 1 "ford pinto"
20.0 4 130.0 102.0 3150. 15.7 76 2 "volvo 245"
13.0 8 318.0 150.0 3940. 13.2 76 1 "plymouth volare premier v8"
19.0 4 120.0 88.00 3270. 21.9 76 2 "peugeot 504"
19.0 6 156.0 108.0 2930. 15.5 76 3 "toyota mark ii"
16.5 6 168.0 120.0 3820. 16.7 76 2 "mercedes-benz 280s"
16.5 8 350.0 180.0 4380. 12.1 76 1 "cadillac seville"
13.0 8 350.0 145.0 4055. 12.0 76 1 "chevy c10"
13.0 8 302.0 130.0 3870. 15.0 76 1 "ford f108"
13.0 8 318.0 150.0 3755. 14.0 76 1 "dodge d100"
31.5 4 98.00 68.00 2045. 18.5 77 3 "honda accord cvcc"
30.0 4 111.0 80.00 2155. 14.8 77 1 "buick opel isuzu deluxe"
36.0 4 79.00 58.00 1825. 18.6 77 2 "renault 5 gtl"
25.5 4 122.0 96.00 2300. 15.5 77 1 "plymouth arrow gs"
33.5 4 85.00 70.00 1945. 16.8 77 3 "datsun f-10 hatchback"
17.5 8 305.0 145.0 3880. 12.5 77 1 "chevrolet caprice classic"
17.0 8 260.0 110.0 4060. 19.0 77 1 "oldsmobile cutlass supreme"
15.5 8 318.0 145.0 4140. 13.7 77 1 "dodge monaco brougham"
15.0 8 302.0 130.0 4295. 14.9 77 1 "mercury cougar brougham"
17.5 6 250.0 110.0 3520. 16.4 77 1 "chevrolet concours"
20.5 6 231.0 105.0 3425. 16.9 77 1 "buick skylark"
19.0 6 225.0 100.0 3630. 17.7 77 1 "plymouth volare custom"
18.5 6 250.0 98.00 3525. 19.0 77 1 "ford granada"
16.0 8 400.0 180.0 4220. 11.1 77 1 "pontiac grand prix lj"
15.5 8 350.0 170.0 4165. 11.4 77 1 "chevrolet monte carlo landau"
15.5 8 400.0 190.0 4325. 12.2 77 1 "chrysler cordoba"
16.0 8 351.0 149.0 4335. 14.5 77 1 "ford thunderbird"
29.0 4 97.00 78.00 1940. 14.5 77 2 "volkswagen rabbit custom"
24.5 4 151.0 88.00 2740. 16.0 77 1 "pontiac sunbird coupe"
26.0 4 97.00 75.00 2265. 18.2 77 3 "toyota corolla liftback"
25.5 4 140.0 89.00 2755. 15.8 77 1 "ford mustang ii 2+2"
30.5 4 98.00 63.00 2051. 17.0 77 1 "chevrolet chevette"
33.5 4 98.00 83.00 2075. 15.9 77 1 "dodge colt m/m"
30.0 4 97.00 67.00 1985. 16.4 77 3 "subaru dl"
30.5 4 97.00 78.00 2190. 14.1 77 2 "volkswagen dasher"
22.0 6 146.0 97.00 2815. 14.5 77 3 "datsun 810"
21.5 4 121.0 110.0 2600. 12.8 77 2 "bmw 320i"
21.5 3 80.00 110.0 2720. 13.5 77 3 "mazda rx-4"
43.1 4 90.00 48.00 1985. 21.5 78 2 "volkswagen rabbit custom diesel"
36.1 4 98.00 66.00 1800. 14.4 78 1 "ford fiesta"
32.8 4 78.00 52.00 1985. 19.4 78 3 "mazda glc deluxe"
39.4 4 85.00 70.00 2070. 18.6 78 3 "datsun b210 gx"
36.1 4 91.00 60.00 1800. 16.4 78 3 "honda civic cvcc"
19.9 8 260.0 110.0 3365. 15.5 78 1 "oldsmobile cutlass salon brougham"
19.4 8 318.0 140.0 3735. 13.2 78 1 "dodge diplomat"
20.2 8 302.0 139.0 3570. 12.8 78 1 "mercury monarch ghia"
19.2 6 231.0 105.0 3535. 19.2 78 1 "pontiac phoenix lj"
20.5 6 200.0 95.00 3155. 18.2 78 1 "chevrolet malibu"
20.2 6 200.0 85.00 2965. 15.8 78 1 "ford fairmont (auto)"
25.1 4 140.0 88.00 2720. 15.4 78 1 "ford fairmont (man)"
20.5 6 225.0 100.0 3430. 17.2 78 1 "plymouth volare"
19.4 6 232.0 90.00 3210. 17.2 78 1 "amc concord"
20.6 6 231.0 105.0 3380. 15.8 78 1 "buick century special"
20.8 6 200.0 85.00 3070. 16.7 78 1 "mercury zephyr"
18.6 6 225.0 110.0 3620. 18.7 78 1 "dodge aspen"
18.1 6 258.0 120.0 3410. 15.1 78 1 "amc concord d/l"
19.2 8 305.0 145.0 3425. 13.2 78 1 "chevrolet monte carlo landau"
17.7 6 231.0 165.0 3445. 13.4 78 1 "buick regal sport coupe (turbo)"
18.1 8 302.0 139.0 3205. 11.2 78 1 "ford futura"
17.5 8 318.0 140.0 4080. 13.7 78 1 "dodge magnum xe"
30.0 4 98.00 68.00 2155. 16.5 78 1 "chevrolet chevette"
27.5 4 134.0 95.00 2560. 14.2 78 3 "toyota corona"
27.2 4 119.0 97.00 2300. 14.7 78 3 "datsun 510"
30.9 4 105.0 75.00 2230. 14.5 78 1 "dodge omni"
21.1 4 134.0 95.00 2515. 14.8 78 3 "toyota celica gt liftback"
23.2 4 156.0 105.0 2745. 16.7 78 1 "plymouth sapporo"
23.8 4 151.0 85.00 2855. 17.6 78 1 "oldsmobile starfire sx"
23.9 4 119.0 97.00 2405. 14.9 78 3 "datsun 200-sx"
20.3 5 131.0 103.0 2830. 15.9 78 2 "audi 5000"
17.0 6 163.0 125.0 3140. 13.6 78 2 "volvo 264gl"
21.6 4 121.0 115.0 2795. 15.7 78 2 "saab 99gle"
16.2 6 163.0 133.0 3410. 15.8 78 2 "peugeot 604sl"
31.5 4 89.00 71.00 1990. 14.9 78 2 "volkswagen scirocco"
29.5 4 98.00 68.00 2135. 16.6 78 3 "honda accord lx"
21.5 6 231.0 115.0 3245. 15.4 79 1 "pontiac lemans v6"
19.8 6 200.0 85.00 2990. 18.2 79 1 "mercury zephyr 6"
22.3 4 140.0 88.00 2890. 17.3 79 1 "ford fairmont 4"
20.2 6 232.0 90.00 3265. 18.2 79 1 "amc concord dl 6"
20.6 6 225.0 110.0 3360. 16.6 79 1 "dodge aspen 6"
17.0 8 305.0 130.0 3840. 15.4 79 1 "chevrolet caprice classic"
17.6 8 302.0 129.0 3725. 13.4 79 1 "ford ltd landau"
16.5 8 351.0 138.0 3955. 13.2 79 1 "mercury grand marquis"
18.2 8 318.0 135.0 3830. 15.2 79 1 "dodge st. regis"
16.9 8 350.0 155.0 4360. 14.9 79 1 "buick estate wagon (sw)"
15.5 8 351.0 142.0 4054. 14.3 79 1 "ford country squire (sw)"
19.2 8 267.0 125.0 3605. 15.0 79 1 "chevrolet malibu classic (sw)"
18.5 8 360.0 150.0 3940. 13.0 79 1 "chrysler lebaron town @ country (sw)"
31.9 4 89.00 71.00 1925. 14.0 79 2 "vw rabbit custom"
34.1 4 86.00 65.00 1975. 15.2 79 3 "maxda glc deluxe"
35.7 4 98.00 80.00 1915. 14.4 79 1 "dodge colt hatchback custom"
27.4 4 121.0 80.00 2670. 15.0 79 1 "amc spirit dl"
25.4 5 183.0 77.00 3530. 20.1 79 2 "mercedes benz 300d"
23.0 8 350.0 125.0 3900. 17.4 79 1 "cadillac eldorado"
27.2 4 141.0 71.00 3190. 24.8 79 2 "peugeot 504"
23.9 8 260.0 90.00 3420. 22.2 79 1 "oldsmobile cutlass salon brougham"
34.2 4 105.0 70.00 2200. 13.2 79 1 "plymouth horizon"
34.5 4 105.0 70.00 2150. 14.9 79 1 "plymouth horizon tc3"
31.8 4 85.00 65.00 2020. 19.2 79 3 "datsun 210"
37.3 4 91.00 69.00 2130. 14.7 79 2 "fiat strada custom"
28.4 4 151.0 90.00 2670. 16.0 79 1 "buick skylark limited"
28.8 6 173.0 115.0 2595. 11.3 79 1 "chevrolet citation"
26.8 6 173.0 115.0 2700. 12.9 79 1 "oldsmobile omega brougham"
33.5 4 151.0 90.00 2556. 13.2 79 1 "pontiac phoenix"
41.5 4 98.00 76.00 2144. 14.7 80 2 "vw rabbit"
38.1 4 89.00 60.00 1968. 18.8 80 3 "toyota corolla tercel"
32.1 4 98.00 70.00 2120. 15.5 80 1 "chevrolet chevette"
37.2 4 86.00 65.00 2019. 16.4 80 3 "datsun 310"
28.0 4 151.0 90.00 2678. 16.5 80 1 "chevrolet citation"
26.4 4 140.0 88.00 2870. 18.1 80 1 "ford fairmont"
24.3 4 151.0 90.00 3003. 20.1 80 1 "amc concord"
19.1 6 225.0 90.00 3381. 18.7 80 1 "dodge aspen"
34.3 4 97.00 78.00 2188. 15.8 80 2 "audi 4000"
29.8 4 134.0 90.00 2711. 15.5 80 3 "toyota corona liftback"
31.3 4 120.0 75.00 2542. 17.5 80 3 "mazda 626"
37.0 4 119.0 92.00 2434. 15.0 80 3 "datsun 510 hatchback"
32.2 4 108.0 75.00 2265. 15.2 80 3 "toyota corolla"
46.6 4 86.00 65.00 2110. 17.9 80 3 "mazda glc"
27.9 4 156.0 105.0 2800. 14.4 80 1 "dodge colt"
40.8 4 85.00 65.00 2110. 19.2 80 3 "datsun 210"
44.3 4 90.00 48.00 2085. 21.7 80 2 "vw rabbit c (diesel)"
43.4 4 90.00 48.00 2335. 23.7 80 2 "vw dasher (diesel)"
36.4 5 121.0 67.00 2950. 19.9 80 2 "audi 5000s (diesel)"
30.0 4 146.0 67.00 3250. 21.8 80 2 "mercedes-benz 240d"
44.6 4 91.00 67.00 1850. 13.8 80 3 "honda civic 1500 gl"
40.9 4 85.00 ? 1835. 17.3 80 2 "renault lecar deluxe"
33.8 4 97.00 67.00 2145. 18.0 80 3 "subaru dl"
29.8 4 89.00 62.00 1845. 15.3 80 2 "vokswagen rabbit"
32.7 6 168.0 132.0 2910. 11.4 80 3 "datsun 280-zx"
23.7 3 70.00 100.0 2420. 12.5 80 3 "mazda rx-7 gs"
35.0 4 122.0 88.00 2500. 15.1 80 2 "triumph tr7 coupe"
23.6 4 140.0 ? 2905. 14.3 80 1 "ford mustang cobra"
32.4 4 107.0 72.00 2290. 17.0 80 3 "honda accord"
27.2 4 135.0 84.00 2490. 15.7 81 1 "plymouth reliant"
26.6 4 151.0 84.00 2635. 16.4 81 1 "buick skylark"
25.8 4 156.0 92.00 2620. 14.4 81 1 "dodge aries wagon (sw)"
23.5 6 173.0 110.0 2725. 12.6 81 1 "chevrolet citation"
30.0 4 135.0 84.00 2385. 12.9 81 1 "plymouth reliant"
39.1 4 79.00 58.00 1755. 16.9 81 3 "toyota starlet"
39.0 4 86.00 64.00 1875. 16.4 81 1 "plymouth champ"
35.1 4 81.00 60.00 1760. 16.1 81 3 "honda civic 1300"
32.3 4 97.00 67.00 2065. 17.8 81 3 "subaru"
37.0 4 85.00 65.00 1975. 19.4 81 3 "datsun 210 mpg"
37.7 4 89.00 62.00 2050. 17.3 81 3 "toyota tercel"
34.1 4 91.00 68.00 1985. 16.0 81 3 "mazda glc 4"
34.7 4 105.0 63.00 2215. 14.9 81 1 "plymouth horizon 4"
34.4 4 98.00 65.00 2045. 16.2 81 1 "ford escort 4w"
29.9 4 98.00 65.00 2380. 20.7 81 1 "ford escort 2h"
33.0 4 105.0 74.00 2190. 14.2 81 2 "volkswagen jetta"
34.5 4 100.0 ? 2320. 15.8 81 2 "renault 18i"
33.7 4 107.0 75.00 2210. 14.4 81 3 "honda prelude"
32.4 4 108.0 75.00 2350. 16.8 81 3 "toyota corolla"
32.9 4 119.0 100.0 2615. 14.8 81 3 "datsun 200sx"
31.6 4 120.0 74.00 2635. 18.3 81 3 "mazda 626"
28.1 4 141.0 80.00 3230. 20.4 81 2 "peugeot 505s turbo diesel"
30.7 6 145.0 76.00 3160. 19.6 81 2 "volvo diesel"
25.4 6 168.0 116.0 2900. 12.6 81 3 "toyota cressida"
24.2 6 146.0 120.0 2930. 13.8 81 3 "datsun 810 maxima"
22.4 6 231.0 110.0 3415. 15.8 81 1 "buick century"
26.6 8 350.0 105.0 3725. 19.0 81 1 "oldsmobile cutlass ls"
20.2 6 200.0 88.00 3060. 17.1 81 1 "ford granada gl"
17.6 6 225.0 85.00 3465. 16.6 81 1 "chrysler lebaron salon"
28.0 4 112.0 88.00 2605. 19.6 82 1 "chevrolet cavalier"
27.0 4 112.0 88.00 2640. 18.6 82 1 "chevrolet cavalier wagon"
34.0 4 112.0 88.00 2395. 18.0 82 1 "chevrolet cavalier 2-door"
31.0 4 112.0 85.00 2575. 16.2 82 1 "pontiac j2000 se hatchback"
29.0 4 135.0 84.00 2525. 16.0 82 1 "dodge aries se"
27.0 4 151.0 90.00 2735. 18.0 82 1 "pontiac phoenix"
24.0 4 140.0 92.00 2865. 16.4 82 1 "ford fairmont futura"
23.0 4 151.0 ? 3035. 20.5 82 1 "amc concord dl"
36.0 4 105.0 74.00 1980. 15.3 82 2 "volkswagen rabbit l"
37.0 4 91.00 68.00 2025. 18.2 82 3 "mazda glc custom l"
31.0 4 91.00 68.00 1970. 17.6 82 3 "mazda glc custom"
38.0 4 105.0 63.00 2125. 14.7 82 1 "plymouth horizon miser"
36.0 4 98.00 70.00 2125. 17.3 82 1 "mercury lynx l"
36.0 4 120.0 88.00 2160. 14.5 82 3 "nissan stanza xe"
36.0 4 107.0 75.00 2205. 14.5 82 3 "honda accord"
34.0 4 108.0 70.00 2245 16.9 82 3 "toyota corolla"
38.0 4 91.00 67.00 1965. 15.0 82 3 "honda civic"
32.0 4 91.00 67.00 1965. 15.7 82 3 "honda civic (auto)"
38.0 4 91.00 67.00 1995. 16.2 82 3 "datsun 310 gx"
25.0 6 181.0 110.0 2945. 16.4 82 1 "buick century limited"
38.0 6 262.0 85.00 3015. 17.0 82 1 "oldsmobile cutlass ciera (diesel)"
26.0 4 156.0 92.00 2585. 14.5 82 1 "chrysler lebaron medallion"
22.0 6 232.0 112.0 2835 14.7 82 1 "ford granada l"
32.0 4 144.0 96.00 2665. 13.9 82 3 "toyota celica gt"
36.0 4 135.0 84.00 2370. 13.0 82 1 "dodge charger 2.2"
27.0 4 151.0 90.00 2950. 17.3 82 1 "chevrolet camaro"
27.0 4 140.0 86.00 2790. 15.6 82 1 "ford mustang gl"
44.0 4 97.00 52.00 2130. 24.6 82 2 "vw pickup"
32.0 4 135.0 84.00 2295. 11.6 82 1 "dodge rampage"
28.0 4 120.0 79.00 2625. 18.6 82 1 "ford ranger"
31.0 4 119.0 82.00 2720. 19.4 82 1 "chevy s-10"
We can see that the data seem to be regularly ordered columns of data, each corresponding to the attributes described in auto-mpg.names
.
So how can we extract these columns separately? One way is to manually use string operations to process each line. The loop below shows how one might go about this. I’ve left in the print
and break
statements I used to debug while doing this process.
autoData = []
with open("./Resources/" + mpgData, "r") as f:
tmpData = f.readlines()
for row in tmpData:
row = row.strip().split("\t")
# print(row) ## Once I confirmed that the above function worked
# break ## to remove the "\t" and "\n" I moved on.
newRow = []
for el in row: ## We now have two elements in the row
## And we want to get rid of the spaces.
newRow.append(el.split(" "))
# print(newRow) ## Looking at the output, we see that we split
# break ## the string containing the name of the car
# ## so let's try something else.
## We know that we want to keep the car name intact,
## so only split the first string.
newRow = row[0].split(" ") + [row[1]]
# print(newRow) ## Checking the work...
# break
## Let's get rid of the empty elements and convert the numbers
row = []
for el in newRow[:-1]:
if len(el) > 0:
if el != "?": ## It turns out this data set has missing
## values coded as "?"... That's annoying!
row.append(float(el))
else:
row.append(np.nan) ## The `np.nan` stands for "Not
## a number" and is a float that
## stands for missing values.
row.append(newRow[-1])
# print(row) ## Checking the work...
# break
autoData.append(row)
print(autoData[0])
[18.0, 8.0, 307.0, 130.0, 3504.0, 12.0, 70.0, 1.0, '"chevrolet chevelle malibu"']
As an alternative, we might also want to try and use loader functions like np.loadtxt
or pandas.read_csv
. Unfortunately, this data was stored in a manner that will stymie the np.loadtxt
function, however the more sophisticated function from pandas
can get the job done with a bit of tweaking.
import pandas as pd
colNames = ['mpg', 'cylinders', 'displacement', 'horsepower',
'weight', 'acceleration', 'model_year', 'origin', 'car_name']
autoDF = pd.read_csv("./Resources/" + mpgData,
delim_whitespace=True,
na_values=["?"],
names=colNames)
autoDF.head()
mpg | cylinders | displacement | horsepower | weight | acceleration | model_year | origin | car_name | |
---|---|---|---|---|---|---|---|---|---|
0 | 18.0 | 8 | 307.0 | 130.0 | 3504.0 | 12.0 | 70 | 1 | chevrolet chevelle malibu |
1 | 15.0 | 8 | 350.0 | 165.0 | 3693.0 | 11.5 | 70 | 1 | buick skylark 320 |
2 | 18.0 | 8 | 318.0 | 150.0 | 3436.0 | 11.0 | 70 | 1 | plymouth satellite |
3 | 16.0 | 8 | 304.0 | 150.0 | 3433.0 | 12.0 | 70 | 1 | amc rebel sst |
4 | 17.0 | 8 | 302.0 | 140.0 | 3449.0 | 10.5 | 70 | 1 | ford torino |
Of course all of this was a bit of overkill as we really were only asked to consider the 1st, 4th, and 7th observations. We now know that these are mpg
, horsepower
and model_year
. We can extract these from either our manually loaded data (which is a list of lists) or from the pandas
DataFrame.
data = []
for ii, row in enumerate(autoData):
newRow = [row[0], row[3], row[6]]
if np.nan not in newRow: ## Only add in complete data.
data.append(newRow)
data = np.array(data)
print(f"'{mpgData}' has been loaded and columns extracted. Shape =",
data.shape)
## Alternately, we can extract from the pandas DataFrame
## We use the `dropna` function to drop the missing data and the `values`
## attribute to return the output as an array.
data = autoDF[[colNames[0], colNames[3], colNames[6]]].dropna().values
print(f"'{mpgData}' has been loaded and columns extracted. Shape =",
data.shape)
print(f"After `values` the type of the data is {type(data)}")
'auto-mpg.data' has been loaded and columns extracted. Shape = (392, 3)
'auto-mpg.data' has been loaded and columns extracted. Shape = (392, 3)
After `values` the type of the data is <class 'numpy.ndarray'>
Part 2.#
Report the number of samples in your data set, save it as \(N\), and calculate the mean, median, standard deviation, and IQR (inter-quartile range) of each of the measurements in the data set.
You should be somewhat comfortable with these processes at this point, so instead I am going to illustrate what this might look like if we kept using the DataFrame structure.
dataDF = autoDF[[colNames[0], colNames[3], colNames[6]]].dropna()
dataDF.describe()
mpg | horsepower | model_year | |
---|---|---|---|
count | 392.000000 | 392.000000 | 392.000000 |
mean | 23.445918 | 104.469388 | 75.979592 |
std | 7.805007 | 38.491160 | 3.683737 |
min | 9.000000 | 46.000000 | 70.000000 |
25% | 17.000000 | 75.000000 | 73.000000 |
50% | 22.750000 | 93.500000 | 76.000000 |
75% | 29.000000 | 126.000000 | 79.000000 |
max | 46.600000 | 230.000000 | 82.000000 |
Part 3.#
Use appropriate plots to show how the different quantities depend on each other. If you loaded the data as a pandas.DataFrame
, try using the sns.pairplot
command. Does it seem that the variables depend on each other linearly?
fig, axes = plt.subplots(3, 3, figsize=(12, 8), sharex="col")
_ = axes[0, 0].hist(dataDF.mpg.values, bins=20)
_ = axes[0, 1].scatter(dataDF.horsepower, dataDF.mpg, s=5, alpha=0.7)
_ = axes[0, 2].scatter(dataDF.model_year, dataDF.mpg, s=5, alpha=0.7)
_ = axes[1, 1].hist(dataDF.horsepower.values, bins=20)
_ = axes[1, 0].scatter(dataDF.mpg, dataDF.horsepower, s=5, alpha=0.7)
_ = axes[1, 2].scatter(dataDF.model_year, dataDF.horsepower, s=5, alpha=0.7)
_ = axes[2, 2].hist(dataDF.model_year.values, bins=20)
_ = axes[2, 0].scatter(dataDF.mpg, dataDF.model_year, s=5, alpha=0.7)
_ = axes[2, 1].scatter(dataDF.horsepower, dataDF.model_year, s=5, alpha=0.7)
for ii in range(3):
_ = axes[ii, 0].set_xlabel("MPG")
_ = axes[ii, 1].set_xlabel("Horsepower")
_ = axes[ii, 2].set_xlabel("Model Year")
_ = axes[0, ii].set_ylabel("MPG")
_ = axes[1, ii].set_ylabel("Horsepower")
_ = axes[2, ii].set_ylabel("Model Year")
_ = axes[ii, ii].set_ylabel("Count")
_ = fig.tight_layout()
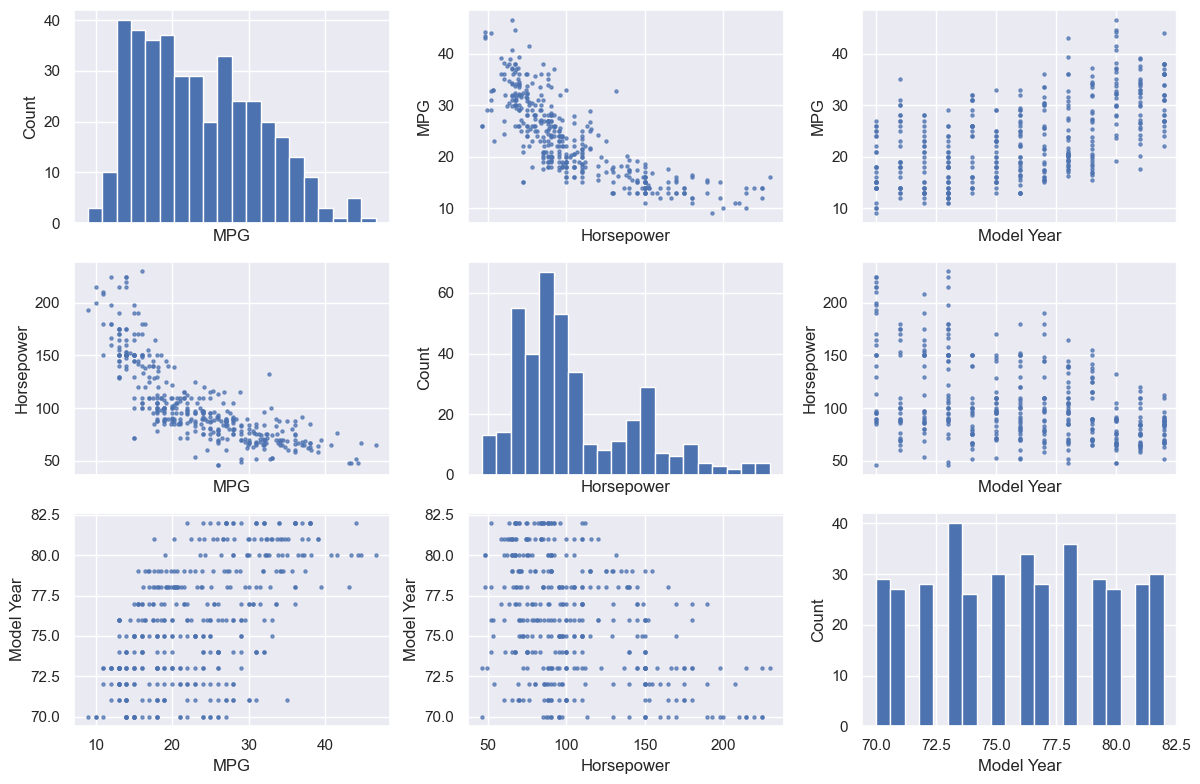
Part 4.#
Use your preferred linear regression package to fit the model. What are the values of \(\beta_0\), \(\beta_3\), and \(\beta_6\)? If you’re using a package that returns this information, what is the confidence interval for each of the regression coefficients?
We’re going to make use of the sklearn.linear_model.LinearRegression
object because we want to do regression with multiple covariates and to show how we can calculate confidence intervals manually.
X = data[:, 1:]
Y = data[:, 0].reshape(-1, 1)
model = skLinReg()
_ = model.fit(X, Y)
print(f"The fitted model is: `MPG` = {model.intercept_[0]:.2f} + {model.coef_[0][0]:.2f} `HP` + {model.coef_[0][1]:.2f} `YEAR`")
The fitted model is: `MPG` = -12.74 + -0.13 `HP` + 0.66 `YEAR`
To get the standard errors in the coefficients, which we need to calculate our MLE confidence intervals, we are going to make use of the fact that the covariance matrix can be calculated as \(\Sigma = \sigma^2(X^TX)^{-1}\), where \(\sigma^2\) is the variance of the Gaussian noise. Unfortunately, we don’t know \(\sigma^2\) a priori, so we have to estimate it using the sample variance, \(\hat{\sigma}^2 = \frac{\text{SSR}}{\text{DoF}}\), where SSR is the sum of the squared residuals and DoF is the degrees of freedom in the data. In this case it is \(\text{DoF} = N - P - 1 = 389\), where \(N\) is the number of samples (rows in the data) and \(P\) is the number of covariates (columns in the data).
Y_pred = model.predict(data[:, 1:])
resids = Y - Y_pred
SSR = np.sum(resids ** 2)
N, P = X.shape
sigmaHat = SSR / (N - P - 1)
We then want to calculate \(\hat{\Sigma} = \hat{\sigma}^2(X^TX)^{-1}\), which we can do after adjusting the covariate matrix a bit. Note here we’re making use of the builtin matrix multiplication operator @
and the np.linalg
package, which is designed to do basic linear algebra operations. The standard errors are then the square roots of the diagonal elements of the covariance matrix.
## We need to add a constant column for the intercept term
X_adj = np.ones((N, P + 1))
X_adj[:, 1:] = X
covarHat = np.linalg.inv(X_adj.T @ X_adj) * sigmaHat
stdErrs = np.diag(covarHat) ** 0.5
print(f"The standard error in the intercept estimate is: {stdErrs[0]:.4g}")
print(f"The standard error in the beta_4 estimate is: {stdErrs[1]:.4g}")
print(f"The standard error in the beta_7 estimate is: {stdErrs[2]:.4g}")
The standard error in the intercept estimate is: 5.349
The standard error in the beta_4 estimate is: 0.006341
The standard error in the beta_7 estimate is: 0.06626
We can compare this to the output from statsmodels
to check our work.
import statsmodels.api as sm
smModel = sm.OLS(Y, X_adj)
smResult = smModel.fit()
print(smResult.summary())
OLS Regression Results
==============================================================================
Dep. Variable: y R-squared: 0.685
Model: OLS Adj. R-squared: 0.684
Method: Least Squares F-statistic: 423.9
Date: Tue, 21 Mar 2023 Prob (F-statistic): 1.94e-98
Time: 18:37:01 Log-Likelihood: -1134.5
No. Observations: 392 AIC: 2275.
Df Residuals: 389 BIC: 2287.
Df Model: 2
Covariance Type: nonrobust
==============================================================================
coef std err t P>|t| [0.025 0.975]
------------------------------------------------------------------------------
const -12.7392 5.349 -2.382 0.018 -23.256 -2.223
x1 -0.1317 0.006 -20.761 0.000 -0.144 -0.119
x2 0.6573 0.066 9.919 0.000 0.527 0.788
==============================================================================
Omnibus: 11.834 Durbin-Watson: 1.054
Prob(Omnibus): 0.003 Jarque-Bera (JB): 12.068
Skew: 0.400 Prob(JB): 0.00240
Kurtosis: 3.316 Cond. No. 3.20e+03
==============================================================================
Notes:
[1] Standard Errors assume that the covariance matrix of the errors is correctly specified.
[2] The condition number is large, 3.2e+03. This might indicate that there are
strong multicollinearity or other numerical problems.
Finally we can calculate the confidence intervals using the formula
where \(t_{p, \nu}\) is the \(p^{\text{th}}\) percentile of the \(t\) distribution with \(\nu\) degrees of freedom, \(\hat{\beta}\) is our ML estimate for the regression coefficient, and \(\hat{\sigma}_{\beta}\) is the estimated standard error in the regression coefficient. Implementing this formula, we get:
betas = np.hstack((model.intercept_, model.coef_[0])) ## hstack "stacks" arrays "H"orizontally
## (there's also a np.vstack)
alpha = 0.05
dof = N - P - 1
t_alpha = st.t.ppf(alpha/2, dof)
CI_beta = np.zeros((3, 2))
CI_beta[:, 0] = betas + t_alpha * stdErrs
CI_beta[:, 1] = betas - t_alpha * stdErrs
for ii, number in enumerate([0, 3, 6]):
print(f"Our estimate for beta_{number} is {betas[ii]:.4g}\t({CI_beta[ii, 0]:.4g}, {CI_beta[ii, 1]:.4g})")
Our estimate for beta_0 is -12.74 (-23.26, -2.223)
Our estimate for beta_3 is -0.1317 (-0.1441, -0.1192)
Our estimate for beta_6 is 0.6573 (0.527, 0.7875)
Part 5.#
Generate a new set of \(N\) samples by bootstrapping your data set with replacement. Run linear regression on this new sample and report the regression coefficients.
Importantly here we don’t want to shuffle columns independently, we want to resample the rows of the original data. This means that we want to draw row indices with replacement. We’ll do this easily with the np.random.randint
function.
Looking ahead, we’re going to do this bootstrapping many times, so I’m going to wrap it all in a nice function.
def bootLinReg(X, Y, verbose=False):
N, P = X.shape
P = P + 1
newIdx = np.random.randint(N, size=(N))
newX, newY = X[newIdx], Y[newIdx]
if verbose:
print(f"The shape of the resampled data is {newX.shape}")
model = skLinReg(fit_intercept=False)
_ = model.fit(newX, newY)
return model
Y = Y.reshape(-1, 1)
X_adj = np.ones((N, P + 1))
X_adj[:, 1:] = X
bootModel = bootLinReg(X_adj, Y, verbose=True)
beta_0, beta_4, beta_7 = bootModel.coef_[0]
print(f"The fitted model is: `MPG` = {beta_0:.2f} + {beta_4:.2f} `HP` + {beta_7:.2f} `YEAR`")
The shape of the resampled data is (392, 3)
The fitted model is: `MPG` = -5.82 + -0.13 `HP` + 0.56 `YEAR`
Part 6.#
Do the previous step \(N_{BOOT}\) times, where \(N_{BOOT}\) is a suitably large number. Present the distributions of regression coefficients. Calculate the 2.5th and 97.5th percentiles of each coefficient. If you found a confidence interval earlier, compare it to these percentiles. Plot the point estimates (part 4) of the regression from the data on these distributions; are they near the median, mean, or mode of the distributions?
N_boot = 10000
Y = data[:, 0].reshape(-1, 1)
X_adj = np.ones((N, P + 1))
X_adj[:, 1:] = data[:, 1:]
betaBoot = np.zeros((N_boot, 3))
for ii in range(N_boot):
bootModel = bootLinReg(X_adj, Y)
betaBoot[ii] = bootModel.coef_[0]
fig, axes = plt.subplots(3, 1, figsize=(12, 10))
cblind = sns.color_palette('colorblind')
for ii, number in enumerate([0, 3, 6]):
ax = axes[ii]
bins = np.linspace(betaBoot[:, ii].min(), betaBoot[:, ii].max(), 51)
_ = ax.hist(betaBoot[:, ii], bins=bins, color=cblind[ii], edgecolor='k', alpha=0.5,
label='Bootstrapped Samples')
est_label = r"$\hat{\beta}_" + f"{number}" + r" = $"
est_label += f"{betas[ii]:.4g}\n[{CI_beta[ii, 0]:.4g}, {CI_beta[ii, 1]:.4g}]"
_ = ax.axvline(betas[ii], color=cblind[ii], lw=3,
label=est_label)
_ = ax.axvline(CI_beta[ii, 0], color=cblind[ii], lw=2, linestyle=':')
_ = ax.axvline(CI_beta[ii, 1], color=cblind[ii], lw=2, linestyle=':')
boot_CI = np.percentile(betaBoot[:, ii], [alpha / 2 * 100, (1 - alpha/2) * 100])
boot_label = f"Bootstrapped Interval:\n[{boot_CI[0]:.4g}, {boot_CI[1]:.4g}]"
_ = ax.axvline(boot_CI[0], color='k', lw=2, linestyle=":", label=boot_label)
_ = ax.axvline(boot_CI[1], color='k', lw=2, linestyle=":")
_ = ax.legend()
_ = ax.set_xlabel(r"$\hat{\beta}_" + f"{number}" + r"$")
_ = ax.set_ylabel("Frequency")
_ = ax.set_yticks([])
_ = fig.tight_layout()
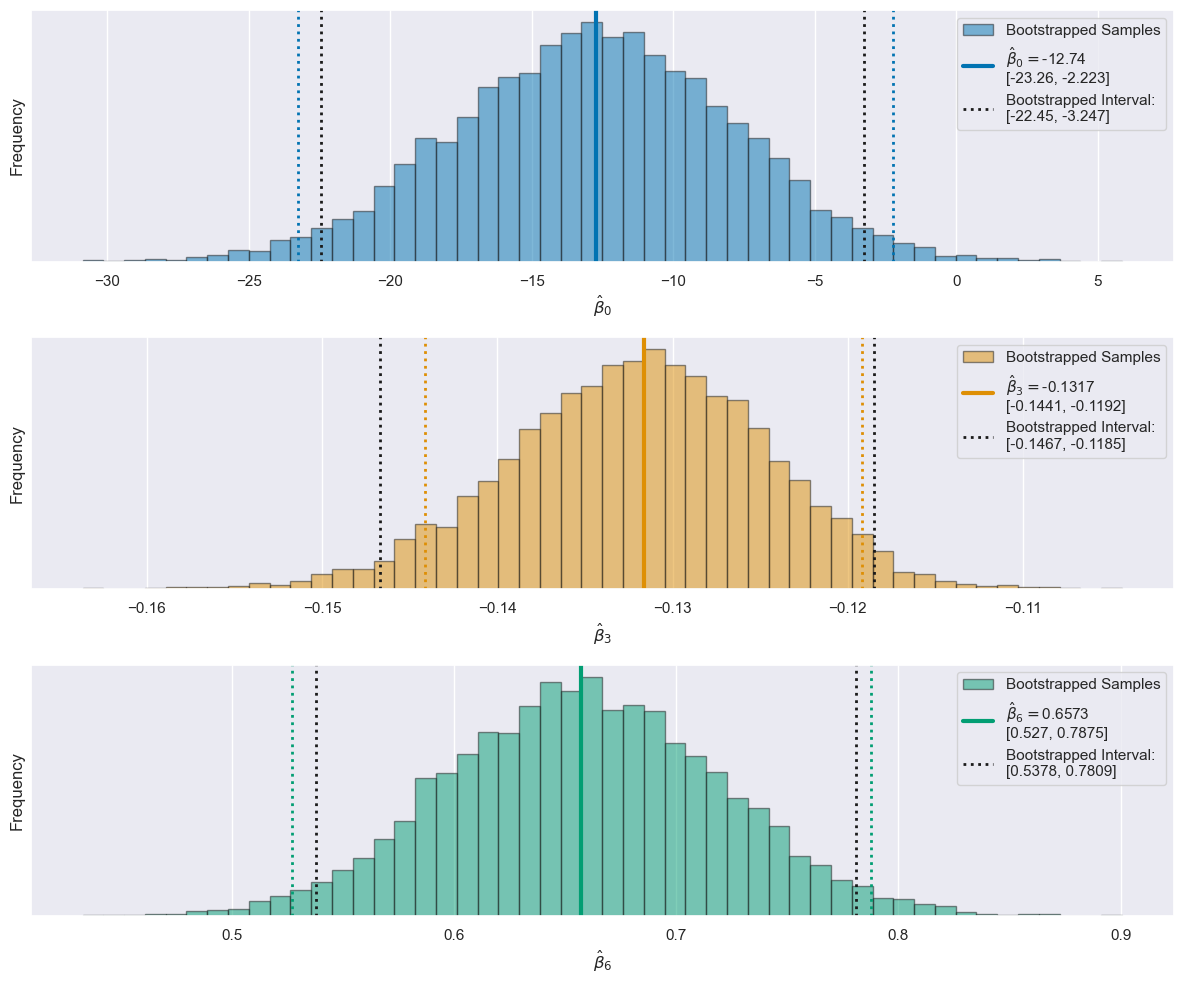